The transportation industry is currently adopting advanced technologies and techniques at a rapid pace. There has been an explosion of firms touting innovative and data-driven insights and a lot of companies offering tools to support predictive analytics, such as AI and machine learning technology. On the one hand, this is very exciting as the widespread use of predictive analytics will play a key role in the advancement of the industry. On the other hand, this technology can be daunting for someone who knows freight and logistics, but doesn’t have a background in mathematics, statistics, or computer science.
To guide you, here are some key questions to ask when considering predictive analytics, machine learning, or AI solutions:
Does your prediction accuracy include true negatives? Or only true positives?
This is the most important question to ask a firm that is predicting something that could be categorized as a yes or a no. For example, will the load in transit be delivered on time? Lots of companies make claims about the accuracy of their predictions. Unfortunately, this doesn’t actually tell you much because there are two ways to be right and two ways to be wrong.
For example, when a load is predicted to be on time and it does arrive on time, this is called a true positive and is the most likely source of an oversimplified claim of accuracy. In order to separate random results from useful predictions, we also must be able to predict the true negatives. So, if a firm predicted that every load would be on time and 86% of the loads arrive on time, this would mean that a true positive was produced 86% of the time with a true negative rate of 0%. In this example, 14% of the loads were not on time, but since the firm’s algorithm predicted that all loads would arrive on time, it did not correctly predict true negatives. A perfect score would accurately predict 86% of the loads on time and 14% not on time. At a minimum, the firm should be able to tell you their ability to predict true positives and true negatives. Even better, they could produce a prediction table like this:
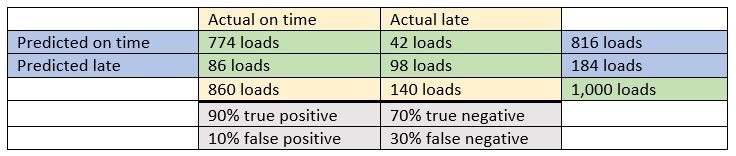
Do your predictions include an out-of-sample measurement?
In general, predictive models use historic data to predict what will happen in the future. To measure how well a model performs, it is critical to test the model using data that was not used to create the model. This requires using out-of-sample data to measure accuracy. Predictive algorithms that are measured without using an out-of-sample approach will almost universally produce inflated results. For example, let’s say that the model is predicting truckload costs in the spot market, and we’re measuring how well the model predicted last week’s spot rates. If last week’s data is included in the data set used by the model, the model sees what it’s predicting within the data it is using. Essentially, the model is “cheating” by getting to see the data that it is supposed to predict. To get a realistic sense of how well the model will perform, it should always be measured using out-of-sample data.
If the firm is measuring something with continuous values like costs, and they present a percent accuracy value, is that value the mean percentage error (MPE) or the mean absolute percentage error (MAPE)?
The last of the three questions may be the most technical but should feel intuitive. Let’s say that the firm is predicting truckload spot rates and says their average error is 1.5%. Sounds pretty good, right? But is it? They are probably reporting their mean percentage error (MPE). This takes all the predictions, compares them to the actuals, converts them into a percentage, and then calculates the average or “mean.” For example, let’s say we have two loads that cost $1,000. If the model shot too high by $500 on one load and then too low by $500 on the next, we would have a positive 50% error and a negative 50% error, which averages to zero. But would you be happy with the $500 error on each load?
You need an additional measure called the mean absolute percentage error (MAPE). This is similar to the MPE, but it doesn’t allow the positive and negative values to cancel each other out. For MAPE, an error is an error regardless of direction. This is a tougher error measure and, in the example above, our MAPE would be 50%.
To fully understand how accurate a model is, you need both values. MPE provides a sense of how centered the model’s predictions are, and MAPE indicates how spread out the errors are. It’s critical to see both values from a firm you’re thinking of working with, not just one.
With the advancement of predictive analytics and other tools, now is an exciting time in the logistics industry. Armed with these questions, you should be one step closer to becoming a savvy consumer of these tools and techniques, and the experts at Echo are always here to help you along the way.
Whether you are a shipper or carrier, Echo’s advanced technology leverages the latest in AI, machine learning, and advanced load matching algorithms to create the most flexible and robust transportation management system available today. Echo’s industry-leading technology, which is built on our cutting-edge architecture, supports rapid deployment of new capabilities and integrates with shipper, carrier, and third-party systems.
To learn more about automation and analytics technology, contact Echo today at 800-354-7993 or info@echo.com.
For informational purposes.